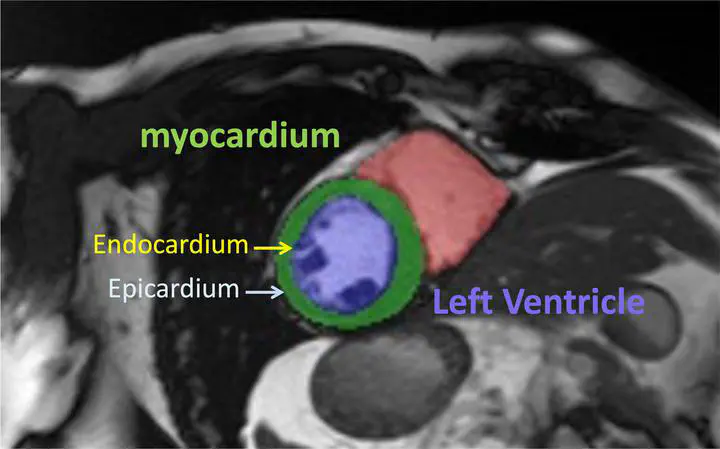
The motion dynamics of the beating heart is a complex process that is closely related to human health. In clinical diagnosis, motion features are important biomarkers in magnetic resonance imaging (MRI) for detecting and monitoring heart disease. They are sensitive to subtle changes in myocardial function and often indicate the early onset of cardiac disease.
The relationship between different areas of the heart evolves over time. This relationship over time can be captured by an irregular graph topology instead of a regular grid.
Before my research, attempts to restructure deep learning architectures to form a grid-like structure to a more irregular framework had not been explored in cardiovascular image analysis.
My study proposes a convolutional neural network architecture that tracks movement spatially and over time at different scales , called a multiscale spatio-temporal graph convolutional network (MST-GCN). This MST-GCN approach is designed to learn the left ventricular (LV) motion patterns from cardiac magnetic resonance image sequences.
Each image is mapped to a network with points called nodes, which are fixed in relation to the heart, but move over time with the movement of the ventricle. This network then extracts features found at different scales and then fuses those together across scales, to model the internal relations of the nodes and form a global representation of the motion of the heart.
We also show that the proposed method can estimate a number of motion-related metrics, including endocardial radii, thickness and strain which are useful for regional LV function assessment.
This technique can take advantage of a sparse representation of the muscular tissue of the heart using contours instead of images.